The last few years have seen a massive acceleration in artificial intelligence. From OpenAI’s ChatGPT to Google’s Gemini and Meta’s LLaMA, large language models (LLMs) are no longer academic novelties—they’re powering enterprise tools, creative assistants, and real-time business automation. But as AI technology evolves rapidly, a key question emerges: can your infrastructure scale with your ambitions?
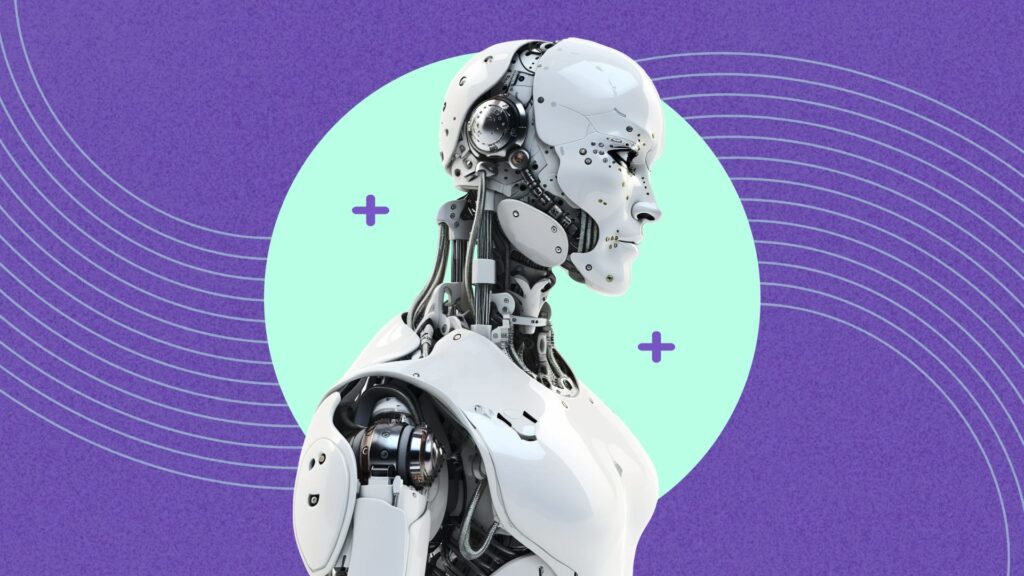
Training AI models is only the beginning. Inference—the act of serving the model and delivering real-time results—is the real test. Whether you’re running customer support chatbots, smart recommendation engines, or predictive analytics, these models are data-hungry, latency-sensitive, and computationally expensive.
This has led companies to rethink their infrastructure stacks. The rise of GPU clusters, dedicated inference hardware, and model optimization frameworks like ONNX or TensorRT shows how important efficient infrastructure has become. AI-ready systems aren’t just about having power—they’re about orchestration, monitoring, and continuous integration.
That’s why they partner with a DevOps team to build and maintain these environments. DevOps teams don’t just provision servers—they design scalable pipelines, automate deployment processes, and enable rollback strategies when things go wrong. More importantly, they ensure that AI models run efficiently in production—without ballooning costs or introducing instability.
As we move toward a future dominated by AI-enhanced workflows, businesses that embrace infrastructure agility will outperform the rest. Your AI solution is only as good as the environment that runs it—and that’s where a capable DevOps partner becomes indispensable.